Unlock the Power of Augmented Analytics: Benefits, Use Cases, and Challenges
Updated on
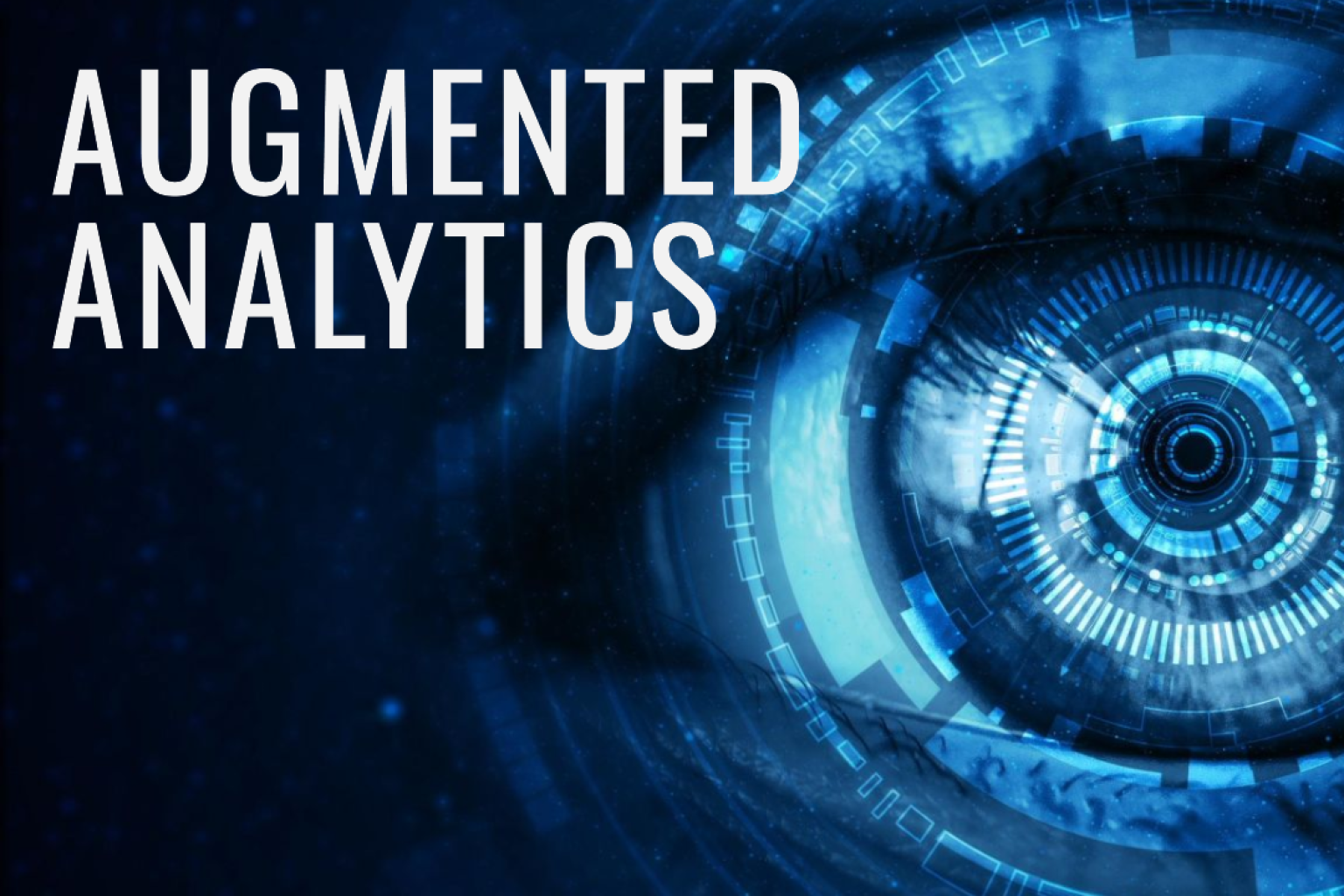
In today's data-driven world, businesses are constantly seeking ways to gain insights from large, complex data sets. Augmented analytics, a powerful technology that combines artificial intelligence (AI) and machine learning (ML) with traditional data analysis, has emerged as a game-changer. This guide will dive into the world of augmented analytics, exploring its benefits, use cases, challenges, and its role in transforming data-driven decision-making.
What is Augmented Analytics?
Augmented analytics leverages AI and ML to enhance data analysis by automating tasks, reducing human bias, and improving the overall accuracy and efficiency of the process. It enables users to quickly and easily explore complex data sets, uncover insights, and make data-driven decisions. This technology is transforming the way organizations approach business intelligence (BI) and analytics, making it more accessible, efficient, and effective.
Benefits of Augmented Analytics
There are numerous benefits to implementing augmented analytics within your organization, including:
- Improved accuracy: AI-powered augmentation reduces human bias and error, leading to more accurate data analysis.
- Increased efficiency: Automation streamlines the data analysis process, allowing users to quickly identify trends and insights.
- Enhanced decision-making: With better access to relevant data, organizations can make more informed, data-driven decisions.
- Greater accessibility: Augmented analytics democratizes data analysis, making it accessible to users with varying skill levels.
- Scalability: Advanced analytics capabilities can be easily scaled to accommodate growing data sets and evolving business needs.
Real-World Use Cases for Augmented Analytics
Augmented analytics is applicable across various industries, providing unique benefits and solutions to specific challenges. Some examples include:
Healthcare
In healthcare, augmented analytics can help providers analyze patient data, predict health outcomes, and optimize treatment plans. By leveraging AI and ML algorithms, healthcare providers can gain insights into patient health, identify trends, and make data-driven decisions to improve patient care.
Finance
Financial institutions can use augmented analytics to detect fraud, manage risk, and optimize investment strategies. By employing AI-powered tools like RATH (opens in a new tab), financial organizations can quickly analyze large volumes of data, uncover hidden patterns and trends, and make data-driven decisions to improve financial performance.
Retail
Retailers can leverage augmented analytics to better understand customer behavior, optimize pricing, and improve supply chain efficiency. By analyzing customer data with AI-powered tools, businesses can identify trends, anticipate customer needs, and develop targeted marketing campaigns to drive customer satisfaction and loyalty.
Marketing
Marketing teams can use augmented analytics to analyze campaign performance, identify trends, and make data-driven decisions to improve ROI. By automating tasks and reducing human bias, augmented analytics enables organizations to make better-informed decisions, leading to improved operational performance and a competitive edge in the market.
Challenges and Best Practices in Implementing Augmented Analytics
Implementing augmented analytics can be challenging due to the complexity of the technology, the need for skilled professionals, and the potential for data privacy and security concerns. To overcome these challenges and maximize the benefits of augmented analytics, organizations should:
- Invest in quality data: Ensure that data is accurate, clean, and well-structured to enable effective analysis.
- Prioritize user training: Provide ongoing training and support to help users understand and make the most of augmented analytics tools.
- Foster a data-driven culture: Encourage collaboration and knowledge-sharing to promote data-driven decision-making across the organization.
- Monitor and maintain data privacy and security: Implement robust data governance policies to protect sensitive information and comply with relevant regulations. 5. Continuously evaluate and optimize: Regularly assess the performance of your augmented analytics tools and processes to ensure continuous improvement.
Augmented Analytics vs. Traditional BI
Augmented analytics differs from traditional BI in several key ways, most notably through its use of AI and ML to enhance data analysis capabilities. Unlike traditional BI, augmented analytics automates tasks, reduces human bias, and provides intuitive data visualization tools, enabling users to interact with data at a contextual level and make better-informed decisions. Additionally, augmented analytics democratizes data analysis, making it accessible to users with varying skill levels, while traditional BI often requires specialized knowledge and expertise.
The Future of Augmented Analytics
The future of augmented analytics is promising, as advances in AI and ML continue to drive innovation and improve the capabilities of data analysis tools. As organizations increasingly adopt augmented analytics, we can expect to see further integration with other technologies, such as the Internet of Things (IoT), predictive analytics, and real-time data processing.
To make the most of augmented analytics, consider exploring platforms like RATH (opens in a new tab), an AI-powered, open-source, automated data analysis and data visualization tool. Discover how RATH's intuitive visualization capabilities can enhance your organization's data analysis processes and drive business success. By staying ahead of the curve and embracing the power of augmented analytics, organizations can unlock valuable insights and make data-driven decisions faster and more efficiently.
With the power of Augmented Analytics engine, RATH makes it very easy to explore and discovert the data patterns and trends. The Data Painter feature is designed to fix this problem. You can easily clean data, model data, and explore data using a Painting Tool, which turns the complex Exploratory Data Analysis process visual and simple.
The following demo video shows the process of finding out the meaning of the trend within a certain data set:
RATH moves even further with the AI Data Visualization capacities that challenges traditional BI tools such as Tableau and PowerBI. The following demo shows how to visualize AirTable data by inserting prompts in natural language.
Imagine having ChatGPT for Data Science! That is what RATH does. You can explore the latest feature right now at RATH (opens in a new tab) website.
Conclusion
In summary, augmented analytics is revolutionizing the way organizations interact with and understand data. By leveraging AI and ML, this powerful technology provides more accurate, efficient, and accessible data analysis capabilities, enabling users to make better-informed decisions. As the future of augmented analytics unfolds, organizations that embrace this technology will be well-positioned to excel in today's increasingly data-driven business landscape. Embrace the potential of augmented analytics and unlock new opportunities for your organization's growth and success.
Frequently Asked Questions
What is the difference between predictive analytics and augmented analytics?
A: Predictive analytics uses statistical modeling and machine learning techniques to analyze historical data and make predictions about future outcomes. Augmented analytics, on the other hand, uses machine learning and artificial intelligence to automate data preparation, analysis, and visualization and provide insights into the data.
Augmented analytics is designed to assist business users in creating and sharing meaningful insights quickly and with little technical knowledge. Unlike traditional analytics software, augmented analytics integrates machine learning to make recommendations and suggest new analysis avenues to users.
What are examples of augmented intelligence?
A: Augmented intelligence is the combination of artificial intelligence and human intelligence working together to produce better outcomes. Here are some examples of augmented intelligence:
-
Personalized recommendations - online retailers and streaming services, such as Amazon and Netflix, use augmented intelligence to analyze customer preferences and activity to provide personalized recommendations.
-
Voice assistants - devices such as Siri and Alexa use natural language processing and machine learning to understand and respond to voice commands.
-
Fraud Detection - augmented intelligence can be used to analyze large data sets and detect fraudulent activities, such as credit card fraud.
What is augmented data intelligence?
A: Augmented data intelligence uses AI and machine learning algorithms to automate data processing, analysis, and visualization. It is designed to help businesses make better decisions by providing insights and data-driven recommendations.
With traditional data analysis, users need to spend a lot of time preparing the data for analysis before gaining any insights. Augmented data intelligence automates much of this process. It can help businesses identify the most important information and generate visualizations with insights that would have otherwise been missed by human analysts.
Who is augmented analytics intended for?
A: Augmented analytics is intended for business users who are not data scientists or analysts but need to leverage insights from large datasets to make informed decisions quickly and accurately.
Augmented analytics allows these users to access the insights they need to make informed decisions, without requiring them to have any technical data expertise. As a result, business executives, marketers, and even customer service representatives can benefit from augmented analytics.
What is augmented intelligence examples?
A: Augmented intelligence examples are designed to improve human decision-making by using AI and machine learning to create more accurate predictions and recommendations. Here are some examples of augmented intelligence:
-
Predictive analytics - uses machine learning to analyze historical data and make predictions about future outcomes.
-
Chatbots - can be programmed to handle common customer requests and queries, freeing up customer service staff to handle more complex requests.
-
Facial recognition - uses AI to analyze and identify photographs and videos.
-
Natural Language Processing (NLP) - uses machine learning and AI to interpret human language, facilitating more advanced and nuanced responses from voice assistants and chatbots.