Casual Analysis or Causal Analysis? Concepts Explained
Updated on
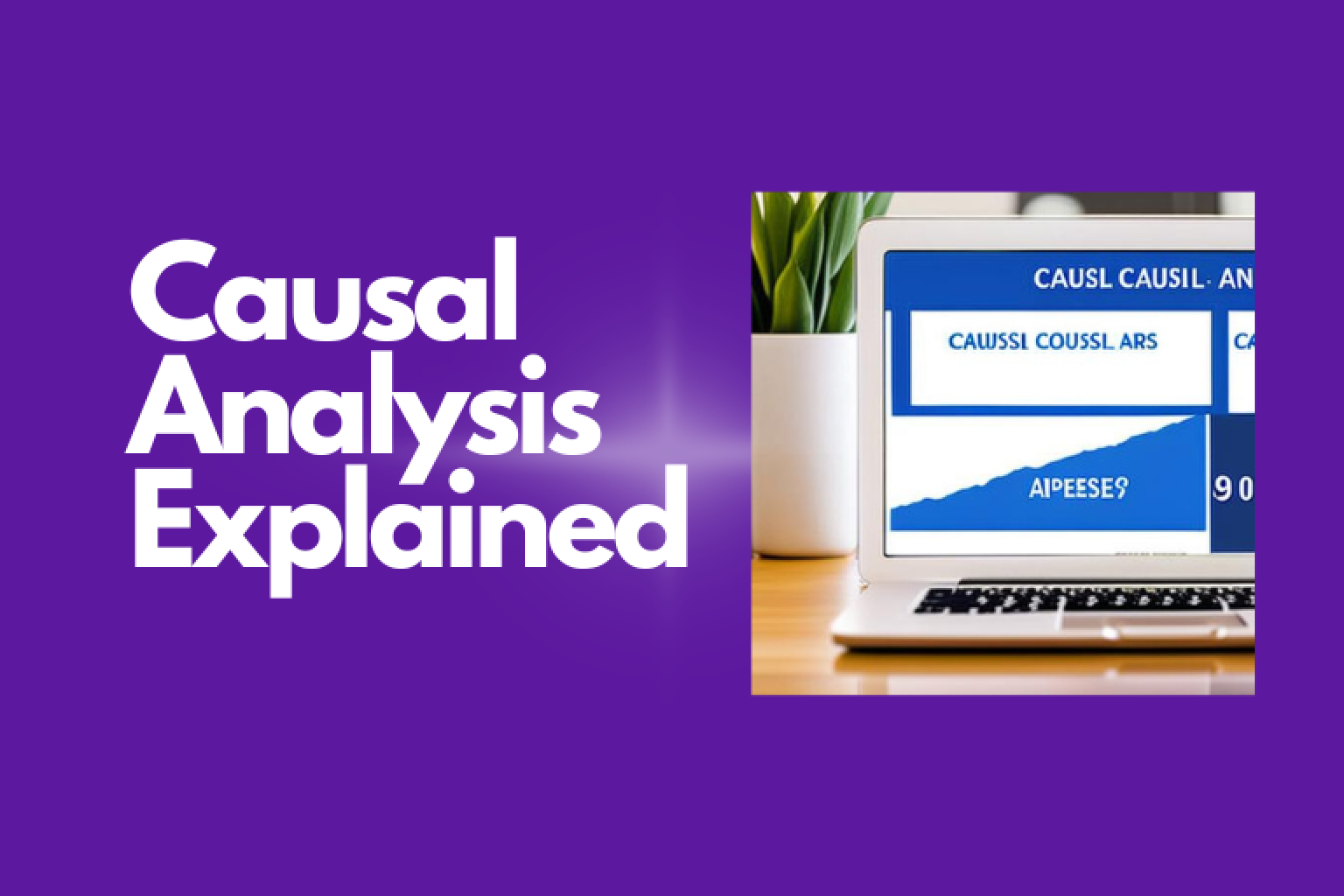
When it comes to understanding the relationships between variables, casual analysis and causal analysis are often confused. While casual analysis refers to the informal examination of data, causal analysis is a more systematic approach to uncovering cause-and-effect relationships, such as Causal Analysis machine learning. In this article, we will delve into the world of causal analysis, exploring various methods, examples, and applications in different fields.
Causal Analysis Methods
There are several methods for conducting causal analysis, ranging from experimental designs to observational studies. Let's take a closer look at each.
Experimental Designs
Experimental designs, such as randomized controlled trials, are considered the gold standard for establishing causality. Researchers manipulate one or more variables while keeping others constant, allowing them to isolate the effects of the manipulated variables. This controlled environment eliminates confounding factors, providing strong evidence for causal relationships.
Observational Studies
Observational studies, on the other hand, rely on statistical techniques like regression analysis, instrumental variables, and propensity score matching to control for confounding factors and uncover causal relationships. These methods are particularly useful when experimental designs are not feasible due to ethical or practical constraints.
Causal Analysis Examples
Causal analysis is employed across various disciplines, such as psychology, economics, and medicine, to identify the underlying causes of observed outcomes. For instance, in psychology, researchers might investigate the impact of childhood experiences on adult behavior. In economics, causal analysis is used to explore the effects of policy changes on economic growth. These examples showcase the widespread use of causal analysis as a tool to understand the world around us.
Causal Analysis in Research
Causal analysis is an essential component of research across various fields. It enables researchers to identify the factors that contribute to specific outcomes, allowing them to develop targeted interventions and make informed decisions. By understanding the underlying causes of observed outcomes, researchers can identify areas for improvement, optimize processes, and ultimately drive progress in their respective fields.
Causal Analysis in Statistics
Statistical techniques play a crucial role in causal analysis, as they help researchers control for confounding variables and establish robust causal relationships. Common statistical methods used in causal analysis include linear regression, logistic regression, and structural equation modeling. These techniques allow researchers to model complex relationships between variables and draw meaningful conclusions from their data.
Causal Analysis in Writing
Causal analysis in writing refers to the systematic exploration and explanation of cause-and-effect relationships in a given subject. This type of writing requires a clear understanding of the underlying factors and mechanisms that lead to specific outcomes. To effectively convey causal relationships, writers must present evidence, consider alternative explanations, and demonstrate a logical progression of ideas.
Causal Analysis in Psychology
In psychology, causal analysis is used to understand the factors that influence human behavior and mental processes. This approach helps researchers identify the underlying mechanisms that drive various psychological phenomena and develop targeted interventions for mental health issues. By uncovering causal relationships, psychologists can better understand the root causes of disorders and provide more effective treatments.
Causal Analysis Python
Python has become a popular choice for causal analysis due to its versatility and extensive library support. Python libraries like Causal Analysis DoWhy, CausalML, and Causalinference provide researchers with a range of tools to perform causal analysis and draw meaningful conclusions from their data.
One powerful alternative for causal analysis is RATH (opens in a new tab), which goes beyond traditional open-source data analysis and visualization tools like Tableau. RATH automates your Exploratory Data Analysis workflow with an Augmented Analytic engine, discovering patterns, insights, and causals. It presents those insights through powerful auto-generated multi-dimensional data visualizations. Learn more about RATH's capabilities by visiting Causal Analysis.
Data Visualization and Causal Analysis
Data visualization is an essential aspect of causal analysis, as it helps researchers communicate complex relationships and findings in an easily digestible format. Tools like Create Data Visualizations can simplify the process of creating visually appealing representations of data, enabling researchers to effectively convey their insights.
When conducting causal analysis, data visualization can reveal hidden patterns, trends, and relationships that may not be immediately apparent through numerical analysis alone. By presenting data in a visually engaging manner, researchers can more easily identify and explore potential causal relationships, facilitating a deeper understanding of the subject matter.
Real-World Applications of Causal Analysis
Causal analysis has far-reaching implications in various sectors, from healthcare and education to finance and public policy. By identifying causal relationships, stakeholders can make better-informed decisions and implement more effective interventions.
Healthcare
In healthcare, causal analysis can help identify the factors that contribute to the onset of diseases, enabling the development of targeted prevention strategies and treatments. By understanding the underlying causes of health issues, medical professionals can better address the needs of their patients and improve overall health outcomes.
Education
In the field of education, causal analysis can inform policymakers and educators about the factors that influence student success. By identifying the causal relationships between educational practices and student outcomes, stakeholders can develop targeted interventions to improve the quality of education and promote equity.
Finance
Causal analysis can also play a crucial role in finance, helping analysts and investors understand the factors that drive market movements and investment performance. By uncovering the underlying causes of financial trends, stakeholders can make more informed decisions and optimize their investment strategies.
Conclusion
In summary, causal analysis is a powerful tool that enables researchers to uncover cause-and-effect relationships in various fields. By employing robust methods and statistical techniques, causal analysis provides valuable insights that inform decision-making and drive innovation. Tools like RATH (opens in a new tab) and Create Data Visualizations further enhance the process by streamlining data analysis and visualization, enabling researchers to communicate their findings effectively. As our understanding of causal relationships continues to grow, so too will our ability to enact meaningful change and drive progress in the world around us.